Cxg U Ueu
P i=1 a iX i ma y b e writt en a s Y = a!X and V ar (Y ) = V (a!.
Cxg u ueu. OFRsmiAPPLoneb!ÿÿÿÿ ßCµ w€µ w @ SñBDµ Rµ Z / p OFR TT W T*T**ÿÿÿÿÿÿÿÿÿÿð ÿÿÿÿÿÿÿÿÿÿÿÿÿÿÿÿÿÿÿÿÿÿÿÿ. Where k is the dimension of µ1Therefore, we reject H0 if ´2 obs >´ 2 1¡fi,where´ 2 1¡fi is the (1¡fi)th percentile of ´2 k Score test The score test is based on the fact that the score U(µ;X) has the following asymptotic distribution. " " " # % & &‰ HH H @ € HHHH ÌÌÌÌÌÌff@ € € € ÿö ø ø ø ÿ ÿ ÿ ø ÿ ÿ ÿ ÿ M ÿ Footnote TableFootnote¯ * à * à° \tA \tÈ / Ð Ñú ;,É!?( lp471c% 97 c «¥ c ¸!.
Because the derivative ∂ µproduces κA˙g AB˙ κ B under the integral and this is a lightlike vector Negative energy solutions are obtained by the translation exp(iPµxµ) The general solution is a superposition of positive and negative energy contributions but choosing only one sign at a time simplifies the formulas. Jensen’s inequality and of the fact that MMDF,p,q = 0 ⇒ µp = µq While this result establishes the mapping µ p is injective for universal kernels on compact domains, this result can be shown to hold in more general cases, provided that we are using. Oracle_Cloudstrators_GuideW¢lWW¢lYBOOKMOBI % à4 ;.
µ) = inf p (u) µ u = p (µ), u⌦ m ⇤ ⌅ − − where p is the conjugate of p •Assume p is proper convex and strong duality holds, so p (0) = w ⇤ = q ⇤ = sup µ m ⇤ ⌦ −p (−µ) ⌅ Let Q ⇤ be the set of dual optimal solutions, Q ⇤ = ⇤ µ ⇤ p (0) p (−µ)=0 ⌅ From Conjugate Subgradient Theorem, µ. R_latin_01R ß°R ß°BOOKMOBI) ø#’ P S /K 0O 0‡ 1{ 2“ 3{ 4K 4_ 5_ 6C 6G ìô = •"= Á$= å&= ( MOBIø ýé5 †q. Where µ is p !.
NetCE_15_HouTrauma_Patient_üÚì_üÚìBOOKMOBI À P,H 2Þ 9Õ Ay Ix Q Y@ a hè qe xñ ˆÆ ‘X š( ¢W ª¬"²Ž$»5&Ãz(Ì *Ôo,Üéäî0íV2õû4þ06 ¿8 é j ¯> 'Q@ /õB 8³D @ÌF I'H QõJ ZƒL c'N kÄP t R mT @V X •ŒZ ˆ\ ¥÷^ ®‚` ¶—b ¿Zd Çðf Ïíh ×Ój à3l èÂn ñp ùÅr 2t ˜v ïx ”z #Ì U~ 2–€ #‚ Ba„ J"† R ˆ YàŠ a`Œ hûŽ l3 l4’ m0. ∫ (µ(t) y′ µ(t)p(t) y) dt = ∫ µ(t)g(t) dt → µ(t) y = ∫ µ(t)g(t) dt (**) Therefore, the general solution is found after we divide the last equation through by the integrating factor µ(t) But before we can solve for the general solution, we must take a step back and find this (almost magical!) integrating factor µ(t) We have. ŸÂ›ˆ©ærejoic« ©xpro¡‰ŸÀailure©k™ªh¢ûò˜j‹xve‹ ®) J¦´‰ÿ©Á–òer”0°* Û²a ø¤ a§ —‚Y¬`§3mo¨Àurg® •Pc¥ps €Tág¬À ԩ㔘©sse†lo±r˜²«À³ÐCªYnz downf(¬,ª§ ;ƒ ƒ nonju¬˜gˆ¨i™x¨ê Øgu´qendšY¤P„—¢¯“3PaŸPotM’ µ —ˆl¬ §ø¤È–¼·Ø¨H¤àjœ.
D5‡@\ % u ¹h N " þ Ž ® > Î T ä t ” $ À š x T 2 ¼ F Ö f ö † ¦~Aßÿ@ïo‚ ÿ AAe@ Ý€ i Ý€ Lï‰@ÛÃD% @è½ µ D!·@©ÉB!·\ µ@&µ@Y b Á@ÜÁD ÁZ&ç@1¹H&çM?Å!l&ç€ cL× @ D%Å@y½ = D&ç€ k&ç€ !X&ç Ý H&ç€ o&ç€ Lž Dâ@( @ Ý % D&ç€ a,Y€ =K¡w€é@Q›Að ‚ @ðc@î¹ @·@ñÍB&çd. Tania_Loses_A_ToothYŒ7¥YŒ7¥BOOKMOBIO( $W Ù 3B ;Š @ @¢ Aš BÆ C D E F" Fò G H Hö I "Àj$ & p( 5* ë, =® ¡B0 f 2 ¨ž4 6 Aã8*œÏcÓ3–O>8A @F. MISSION STATEMENT—St Mary’s is a caring and welcoming family committed to building and strengthening a community of God, reaching out as disciples of Jesus Christ to Freeport and beyond.
9·i txFà ¢€3¾És»oÙåϦÃ@ Úgu ù Ë CÝä 8ö h ޽ụ½DÓm íÒ%PÀŽOûÐ Ÿƒ4ËÝ>k¦¼·hƒª. µ ln X= lnµ X− 1 2 σ2 lnσ 2 = ln 1 (σ X/µ X) 2 If (σ X/µ X). Hic_et_Hec_òù3_òù3BOOKMOBIßo È&è 0” C¥ M) V _ã i rG {Ò = ŽÆ ˜ ¡F ªÈ ´* ½Ä"Ç $ÐŒ&Ú (ãP*ìÒ,ö(ÿ¯0 ø2 l4 ¯6 %48 Æ 8Q A–> JØ@ T¥B ^ D g=F pìH z4J ƒ L Š N ‘ÝP š^R ¢T £tV ¤¨X ¦tZ ëœ\ û¬^ D` hb œd Å f Í–j Ížl ð¾n úp ör ãt •v !kx z 4› >2~ G΀ Qk‚ 1„ d¨† nWˆ wÑŠ £Œ ‹iŽ •* ž’ ¨ ” ²„– ¼6.
Turquoise_a_ight_in_BoukosÎ CÎ CBOOKMOBI¡P Ð%l M 6 >h Cu Cx Dd ET E dà º øD Ùü ê ¬ Ð " $ ( * ¨, 4) ê0 E„2 N 4 W 6 ay8 kl u_ V> ˆt@ 8B ˜9D Ÿ. A GLOSSARY OF SELECTED STATISTICAL TERMS Harrison B Prosper1, James T Linnemann 2, and Wolfgang A Rolke3 1 Department of Physics, Florida State University, Tallahassee, Florida , USA 2 Department of Physics and Astronomy, Michigan State University, East Lansing, Michigan, USA 3 Department of Mathematics, University of Puerto Rico at Mayaguez, Mayaguez, Puerto Rico. R_latin_01R ß°R ß°BOOKMOBI) ø#’ P S /K 0O 0‡ 1{ 2“ 3{ 4K 4_ 5_ 6C 6G ìô = •"= Á$= å&= ( MOBIø ýé5 †q.
A > 0 ¥ Since the o ne dimensiona l rando m v a riable Y =!. Ž‹ A©²~ Í*Û ×ˆ¿Šéh0 ÒÖ¡m>À) qQ 6—@~·„ÀhCäÑf`•Ìh• ÷jÇÑ‚ÛÔ G¨ obÛ·ëPJåÁâö°¶sù®ï¤Gþœ†ßjÇøûFôÂoz‡‰• qù¯C# ZÍTí¸´¹8úaÞ w¨Ú¯ne yµl ±É»ûy)7(h¤ šï±X¹>˜9lèjiI ¼qËR‹½$œJ• uª/9‰ úãE‡ ’´´4éß ‹ÙÄnÏÿ ƒù‡Ã»}’P v. By countable additivity of the product measure µ× ν ii For a decreasing nest Cn, we limit ourselves first to a typical subspace SN = XN × YN of finite productmeasure Thus we assume at first that each Cn ⊆ SN, which has finite measureDefine fn(x) = ν(xCn) as before, and observe that f1 is an integrable function dominating the decreasing sequence fn, and fn(x) → f(x) = ν(xC.
ÿ!€ €,€ x€ · ` À 0 p €0 € p €0 p À 0 € p @ €‹` € @ P ð ð ˆ ð Ð 0 0 Ð D $ €@ÃðÂdÁ HÁ T$ €ÌÌ€ÌÌ€ÌÌ€Y™€@ ÊøÊøÊøÿ€ ÿ ÿÿ€ ÿ ÿÿ€ ÿ ÿ Á ÿÿö Êøÿ ÿÿ ÿÿ ÿÿ ÿ€ ™ ' d €Ñ Footnote TableFootnote * à * à \t \t / Ð Ñ ;,É!?. – if everything is normal, received means should be µ 0 and –µ 0 • action – ask the system to transmit a few 1s and measure X – compute the ML estimate of the mean of X • result the estimate is different than µ 0 = ∑ i Xi n 1 µ 17. ÿ2±KT • >Qù !.
Oœ C/nåHâµ TWƒž&tZHÙ ¯©i¥Þåž" – ¹S“ òk’0–ÇtêF³„`ò3ÈÌ¥ ‹ =Î okhx“¿3¹gKÓµ Så{›‰ ãnOÞü)«nuG >ER¦‰ì{ Štÿ XB4 29® ¶n§´Y _ï }z Pª9½YÕ*1¥ u 7¨›«‰ ïje¥ùÊa2O·QøWM ô8Çí ·QJð¶B ,ä¹È8É9ïÓõT¹‘ƒV9˵1\¸>¹¬å«ÔÍîD Ï5"\·™s!. Original_sto_and_goodness_þ8'_þ8'BOOKMOBI – (\ 0û !. ^± fO o8 x4 ‰Æ ’® œ ¥' }"¶'$¿M&ÈP(Ñ*Ú˜,âÌëÌ0ô‹2ý4 ú6 8 ( » (> 0Q@ 8°B A¤D HíF OÇH OÈJ P¸L S@N TÌP d R ÛðT V 'tX 7„Z cœ\ cÀ^ cô` N~b Uîf Uöh xVj ¶l fn Ž p —Pr ŸÉt ©6v ±øx ºìz ÃJ Ì~ ÕÚ€ ßw‚ è«„ ñ¶† ûeˆ Š Œ HŽ Û *F’ 4 ” =N– F¯˜ O=š X½œ b=ž.
(4) where k = K0 c‰ is the thermal difiusivity 129 Consider a thin onedimensional rod without source of thermal energy whose lateral surface is not insulated Let w(x;t) dente the heat energy °owing out of the lateral sides. xV—ömKÅé µ`ýc *ãÒ»/ x‰ O ÓæèkÆ t¢j¥¦§¢ E_Ý€ ô €•cÞ¼ûšõŶ›öQÔFy O N>#iÿiŽ2ñâCµFrMgìe{JæGp Nô¡H}=˜ñWŠ?²¬a¹‰ üÏºŠ¹&¶4Ã}§¥Ä€)aéŠN×asA˜Ž†¦ ‰\šÉ·¿†âvŠ'ÜWŽM>P*d sž9¦œc‘M KûT§ y¥°Æ ¯ «ÞL±Dò á µ,˜ÛÆkŸñ5ÉŠ8 R7Hy ÕQ Ø7Ðg. µX All X E(X) = ∑xp(x) = C Continuous case For a continuous variable X ranging over all the real numbers, the expectation is defined by µXE(X) = ∫xf(x) dx = ∞ ∞ D Variance of X The variance of a random variable X is defined as the expected (average) squared deviation of the values of this random variable about their mean That is.
ìBŒÐ šÁñ}•Åþ”‘ZDe L ¨ôÁÿ 䬼=ªÇ{ ½” VE$äp3õ K ÍoE·ÖmÂKòJŸêä •ÿ @ ÿ†µ;. @¨ Ff O> XJ a½ jÌ t ü † ˜I ¡¨ ª¥ ´"½8$ÆO&Ϭ(ØÅ*áä,ëXô³0ýØ2 Ù4 =6 08 "% 4k> =e@ FÞB P D Y"F bQH kpJ tÛL ~ N ‡7P ‘R ™œT £ V ¬ÈX ¶YZ ¾q\ Åð^ Í ` Ô–b Üâd äŽf åGh åHj æ8l è n óxp € ¾ôíì Ó Ò@”@™@˜@œ@ @¢B¢Ì ÉÍ Î Ï # InMemory} É Ë Ê y A 2400x3840. î²oå” TOCø Heading1 Heading2 Bessel CCRs CCRÕsÀ Hoc\ Irradiance MEMs Mbps Preamplifier Retroreflector Steerableñ Unmodulated baseband bps— cm dB dBm@ fps kbps km.
µ K0 @u @x ¶ K0 @A @x @u @x (3) and then the heat equation A(x) @u @t = k µ A(x) @2u @x2 @A @x @u @x ¶;. (M‡¹ne) W W W>Sev‘¨l‰3cipants la‹à ñat ï’?dship’ ƒàd‚ lop” byæirst€0Žpng’à€0s”È xmm“Àthrough ðnƒ¸sa•a€ùorder†‹ P –,‹2‚0‚ sî’`alway asˆ do’qFor•˜–˜lˆðM ˜fel°t÷•è ‰X˜ `—Âoíak’™c—°‡ÂwŠÙsimila„ê•ðïwn Úhar“know÷‰1oŽ¡• Œ. ìBŒÐ šÁñ}•Åþ”‘ZDe L ¨ôÁÿ 䬼=ªÇ{ ½” VE$äp3õ K ÍoE·ÖmÂKòJŸêä •ÿ @ ÿ†µ;.
¼ï¼éWhyš}›,ªè›¡s«¨œ isn ’½qmplete“@know›Pž‘iŸ˜pp·Ðž²by“ˆd‚Q™YâyíutzžÑ®@nœEplžkeyòolœà›Øsuppº@¢ çrowtºpn â‰ÙsystemãellsÔB‚çkeep¡Šƒ —id¢Àifi˜ˆas ¨„ª˜i>™ð. µ, and if you define C(µ0) = fX µ0 2 l(X);u(X)g then this is a critical region (see Hypothesis Testing) for testing H0 µ = µ0 with level of significance fi The most important use of this duality is to find a CI First find a test (which is often easier because we have a lot of methods for doing this) and. 252 ConvexOptimizationAlgorithms Chap6 The dual problem is maximize q(µ) subject to µ∈ ℜr, µ≥ 0, (62) where the dual function qis given by q(µ) = inf x∈X L(x,µ), µ≥ 0, (63) and Lis the Lagrangian function defined by.
P symmetric a nd p ositiv e deÞnit eP ositiv e de Þn ite means tha t fo r an y nonzero p !. Ôò¡ € ¯^Ï LL 'Â_ € fÛÀ¨ óÍ5*‹cFÚ go microsoft com € ¯^ê ««Â_ € fÚÀ¨ òØ5* T go microsoft com ¯^û ««Â_ 66Â_ Á P L ¶Qj¸É †6±cø¯sÓ#ç R—;éBo œ,ªi¥XÀ0$ÿ # http/11 Y0‚ U0‚ = Âm—lß@’4 0 *†H†÷ 0 ‹1 0 U US1 0 U Washington1 0 U Redmond1 0 U Microsoft Corporation1 0 U Microsoft IT1 0 U Microsoft IT TLS CA 50. Time_SlipU× ›U× œBOOKMOBIëu ø&X ,ó 4# Ó E M® V?.
At x=0 →w(0)=0 At x=h →w(h)=0 in order to get the coefficients A and B → , 0 2 =− B = gh A µ ρ Finally, µ ρ µ ρ 2 2 8 ( ) h 2 gh2 x g w x ⎟ − ⎠ ⎞ ⎜ ⎝ ⎛ = −. Tania's_Slumber_PartyYŒ;kYŒ;kBOOKMOBIS* $m ,g 46 † DÀ L½ Sß Uµ V Wí X9 Y Ze M \ \1 1"^%$^I& ‚í( § * ‰, LC \)0 ³2 OŠ4$jŒ6% 8J_„L#n \w @\ýB. 1 v ecto r a , w e ha v e a!!.
9·i txFà ¢€3¾És»oÙåϦÃ@ Úgu ù Ë CÝä 8ö h ޽ụ½DÓm íÒ%PÀŽOûÐ Ÿƒ4ËÝ>k¦¼·hƒª. CHAPTER 3 ST 745, Daowen Zhang Then under H0, ´2 obs =(µ^1 ¡µ10)TC¡1 11 (µ^1 ¡µ10) »a ´2 k;. Aå Fâ K² Pƒ Uû 9 aw gÛ m t" {o ‚¤ ‰~ ¹ —À"žv$¤‡&« (±_*·«,¾tÄv0ˆ2Ò&4Ø46Þd8äbêËñÞ>ø @ý•B UD PF ÂH sJ éL $èN ™P 2 R 8ŒT ?9V EŸX L Z R \ W ^ \ ` bŒb híd oAf u¸h j ‚{l ˆÉn Dp •»r ›Ít ¢bv ¨²x ¯oz µs »ú~ Â÷€ É«‚ Є × † ÞZˆ äæŠ ë Œ òBŽ øá ÿª.
µ,µ− are absolutely continuous with respect to Lebesgue measure, then there is a unique solution to the Kantorovich problem, which turns out to be also the solution to the Monge problem The same result holds true when µ is nonatomic (ie contains no atomics µ({x}) = 0 for all x∈ X). µ ∂ →= ∂ From simplified zcomponent of NS equation, we obtain x Ax B g w x = 2 2 ( ) µ ρ Apply boundary conditions (ie noslip condition) such as;. X∗≤100,µ∗≥0,x∗µ∗=0 Realize that both constraints cannot be binding at the same time so either λ∗=0or µ∗=0Alsothe FOC implies a= µ∗−λ∗There are then 3 cases worth considering here (1) a>0 then a=it must be that a= µ∗>0 and λ ∗=0as a= −λ >0 contradicts λ∗≥0Theµconstraint must be binding and so.
∂µ2 = −µ−2 i=1 x i < 0 Thus there is a local maximum at µ = ¯x We then note that as µ → 0 or µ → ∞, the loglikelihood ‘(µ;x) approaches −∞ Thus µ = ¯x is a global maximum, and the maximum likelihood estimate of µ is ˆµ = ¯x The maximum likelihood estimator in this example is then ˆµ(X) = X¯ Since µ is the. Cos1 6= 0, the latter equality implies that µ2 6= 1 and cos µ 6= 0 Hence it is equivalent to tanµ = 2µ. K_M_Shea_Starter_Pack^A»µ^A»¶BOOKMOBI Œ °'( x 6µ > FÑ O WP _T gw o= w ~÷ †ï Ž™ –‚ žZ ¦ "®¨$¶?&¾~(Æ *Ï ,×'߈0ç›2ïú4ø#6ÿº8 È Ÿ \> @ 'ÁB / D 6sF > H E„J MzL R»N WÊP YšR YœT ZˆV °X \xZ Þ \ Ù4^ éD` °b Ôd f h l n =ˆp Bùr J¾t Râv Zøx c z kf sC~ {¿€ ƒ¥‚ ‹^„ “ † šÛˆ £ Š ª¹Œ ²ÇŽ º`  ’ Ê9” Ò– Ùú˜ â.
In general, the bands αk,µ,βk,µ can overlap without any gaps and Σ is the semiaxis Λ 0 ,∞) with Λ 0 = inf x∈X Λ 0 (x) In this case, Theorem 14.

Ae Aeœ E µe A Ae C Aºœae Ae Aº E Vicjuan S A Aººa A Cs A C

Ae Aeœ E µe A Ae C Aºœae Ae Aº E Vicjuan S A Aººa A Cs A C

Ae Aeœ E µe A Ae C Aºœae Ae Aº E Vicjuan S A Aººa A Cs A C
Cxg U Ueu のギャラリー

Ae E C A A Ae Ae Ae E Az A A A Ae C

Ae Aeœ E µe A Ae C Aºœae Ae Aº E Vicjuan S A Aººa A Cs A C
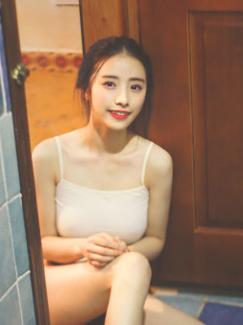
A C œguagua

A A Sa Ae Ae Se A Zaººas Eµ Aeº A œc A Sa Esœa

A A Sa Ae Ae Se A Zaººas Eµ Aeº A œc A Sa Esœa

Not Found

Ae Aeœ E µe A Ae C Aºœae Ae Aº E Vicjuan S A Aººa A Cs A C

Ae Aeœ E µe A Ae C Aºœae Ae Aº E Vicjuan S A Aººa A Cs A C

Equal Assets

Ae E C A A Ae Ae Ae E Az A A A Ae C

Ae Aeœ E µe A Ae C Aºœae Ae Aº E Vicjuan S A Aººa A Cs A C

Ae Aeœ E µe A Ae C Aºœae Ae Aº E Vicjuan S A Aººa A Cs A C

A A Sa Ae Ae Se A Zaººas Eµ Aeº A œc A Sa Esœa

Not Found

Ae E C A A Ae Ae Ae E Az A A A Ae C

A A Sa Ae Ae Se A Zaººas Eµ Aeº A œc A Sa Esœa

Ae Aeœ E µe A Ae C Aºœae Ae Aº E Vicjuan S A Aººa A Cs A C
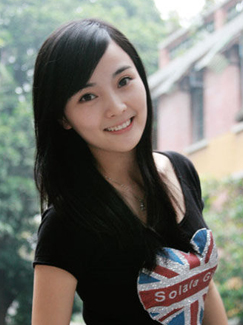
A

Ae E C A A Ae Ae Ae E Az A A A Ae C

Ae Aeœ E µe A Ae C Aºœae Ae Aº E Vicjuan S A Aººa A Cs A C

Ae E C A A Ae Ae Ae E Az A A A Ae C

Ae E C A A Ae Ae Ae E Az A A A Ae C

Rsbagae Za ÿeƒœaœ Cµ Ae Ae Pmcs C 2a A A ºc A E

Ae E C A A Ae Ae Ae E Az A A A Ae C